The 10th Israel Machine Vision Conference (IMVC) took place on March 18, 2019 at Pavilion 10, EXPO Tel Aviv.
Ilan Kadar spoke at a conference on “Computational Photography on Google’s Smartphones”.
Abstract:
The robustness of end-to-end driving policy models depends on having access to the largest possible training dataset, exposing the true diversity of the 10 trillion miles that humans drive every year in the real world. However, current approaches are limited to models trained using homogenous data from a small number of vehicles running in controlled environments or in simulation, which fail to perform adequately in real-world dangerous corner cases. Safe driving requires continuously resolving a long tail of those corner cases. The only possible way to train a robust driving policy model is, therefore, to continuously capture as many of these cases as possible. The capture of driving data is unfortunately constrained by the reduced compute capabilities of the devices running at the edge and the limited network connectivity to the cloud, making the task of building robust end-to-end driving policies very complex.
In this talk, I will give an overview of a network of connected devices deployed at the edge running deep learning models that continuously capture, select, and transfer to the cloud “interesting” monocular camera observations, vehicle motion, and driver actions. The collected data is used to train an end-to-end vehicle driving policy, which also guarantees that the information gain of the learned model is monotonically increasing, effectively becoming progressively more selective of the data captured by the edge devices as it walks down the tail of corner cases.
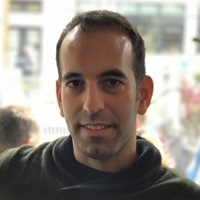
Bio:
Ilan Kadar is the Director of Deep Learning at Nexar. Ilan is responsible for leading the deep learning team and effort to leverage Nexar’s large-scale datasets of real-world driving environments to automotive safety applications. Prior to Nexar, Ilan was leading the image recognition group at Cortica and was responsible for building the company’s machine vision technology. Ilan received his BSc, MSc and PhD degrees in computer science from the Ben-Gurion University of the Negev, Israel, in 2006, 2008, and 2012 respectively (Summa Cum Laude).
His research thesis focused on machine learning algorithms for scene recognition and image retrieval and was published in leading conferences and journals in the areas of machine vision.
For Ilan Kadar’s presentation click here
Legal Disclaimer:
You understand that when using the Site you may be exposed to content from a variety of sources, and that SagivTech is not responsible for the accuracy, usefulness, safety or intellectual property rights of, or relating to, such content and that such content does not express SagivTech’s opinion or endorsement of any subject matter and should not be relied upon as such. SagivTech and its affiliates accept no responsibility for any consequences whatsoever arising from use of such content. You acknowledge that any use of the content is at your own risk.
Leave A Comment