The 10th Israel Machine Vision Conference (IMVC) took place on March 18, 2019 at Pavilion 10, EXPO Tel Aviv.
Elad Richardson spoke at a conference on “You Only Scale Once – Efficient Text Detection using Adaptive Scaling”.
Abstract:
Text detection and recognition systems have gained a significant amount of attention in recent years. Current state-of-art text detection algorithms tackle challenging text instances in natural images. In particular the problem of detecting multi-scale text in a single image still presents a challenge. A common paradigm for dealing with that challenge is simply, given a single-scale text detection algorithm, to re-run that algorithm on different rescaled versions of the original image. While this approach usually achieves a boost in results, it is wasteful and significantly increases runtime.
In our work, we present an approach that bypasses the need to re-run the same detection algorithm on multiple scales. We show that using a simple plug-and-play change in the architecture, we are able to transform a text segmentation Convolutional Neural Network to also detect text scales. Knowing the text scales allows us to adaptively re-scale text regions, and aggregate them into a compact image, which enables our network to detect the smaller text using only one additional pass. We present some qualitative and quantitative results on the ICDAR benchmark, showing that our approach offers a good trade-off between runtime and accuracy.
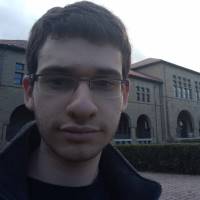
Bio:
Elad Richardson is a Computer Vision enthusiast, focusing on the application of Deep Learning methods for a variety of problems.
Elad completed his M.Sc in Computer Science under the supervision of Prof. Ron Kimmel at the GIP Lab, Technion. His research focused on the applications of neural networks for learning 3D facial reconstructions and was presented at various international conferences. Currently, Elad is a researcher at the Defence Community.
For Elad Richardson’s presentation click here
Legal Disclaimer:
You understand that when using the Site you may be exposed to content from a variety of sources, and that SagivTech is not responsible for the accuracy, usefulness, safety or intellectual property rights of, or relating to, such content and that such content does not express SagivTech’s opinion or endorsement of any subject matter and should not be relied upon as such. SagivTech and its affiliates accept no responsibility for any consequences whatsoever arising from use of such content. You acknowledge that any use of the content is at your own risk.
Leave A Comment