The annual Israel Machine Vision Conference (IMVC) took place on March 28, 2017 at David InterContinental Tel Aviv.
Eran Goldman spoke at a conference on “Employing Context for Fine-Grained Object Recognition in Crowded Images”.
Abstract:
Trax is built to revolutionize the retail industry using cutting edge computer vision techniques. Our challenges include fine-grained visual recognition of densely arranged items in store displays.
In this presentation, we will introduce a novel CNN architecture which learns both local visual features and neighboring class representations, and extends the softmax function to define a probabilistic graphical model.
In test time, the graph is extracted from a context-enhanced detector, which converges into the correct localizations by iterative reduction of the attention regions, and refinement of object-proposal granularity.
The detected items are then classified by the marginal distributions of the graph joint probability, integrating local and spatial features.
We will further demonstrate how the algorithms can be optimized by dynamic programming techniques and parallel pipeline design.
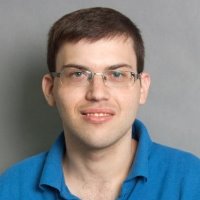
Bio:
Eran Goldman is a Computer Vision Researcher at Trax Image Recognition, developing machine learning algorithms for fine-grained image recognition.
His team is focused on the development of a scalable recognition system by using deep learning technology.
Eran works toward a M.Sc. thesis under the supervision of Prof. Jacob Goldberger from the Bar-Ilan University. His research deals with contextual models for object classification with conditional random field as deep neural network.
For Eran Goldman’s presentation click here
Legal Disclaimer:
You understand that when using the Site you may be exposed to content from a variety of sources, and that SagivTech is not responsible for the accuracy, usefulness, safety or intellectual property rights of, or relating to, such content and that such content does not express SagivTech’s opinion or endorsement of any subject matter and should not be relied upon as such. SagivTech and its affiliates accept no responsibility for any consequences whatsoever arising from use of such content. You acknowledge that any use of the content is at your own risk.
Leave A Comment